| Japanese | English |
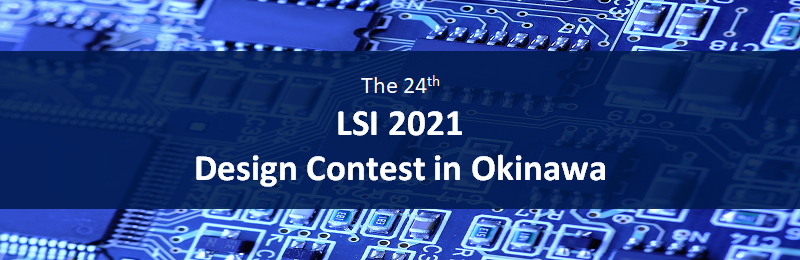
24th LSI Design Contests-in Okinawa Design Specification - 4-1
4-1.Q table
It can be rephrased that the purpose of reinforcement learning is to update the Q value, which is an index of the value of an action, and to complete a table of Q values that will ultimately maximize the reward that an agent can get. Table 1 shows the Q value table of the unlearned state in the example.
*The initial value is generated randomly, and S 25 is set to 0 because it becomes a goal, and there is no need to act further.
Table 1 : Q value table of unlearned status
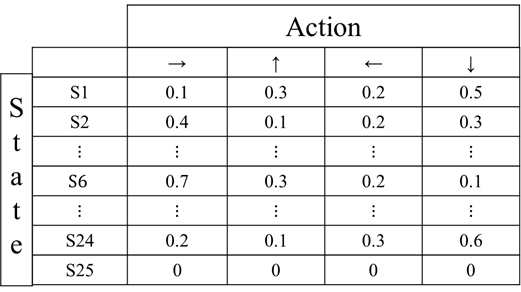
Table 1 quantifies the value of each action in each state. For example:
In S1, the values of "→", "↑", "←", and "↓" are 0.1, 0.3, 0.2, and 0.5, respectively.
It turns out that the most valuable action is to go to the "down arrow.".
In this example, the Q ‐ value table is completed through reinforcement learning.